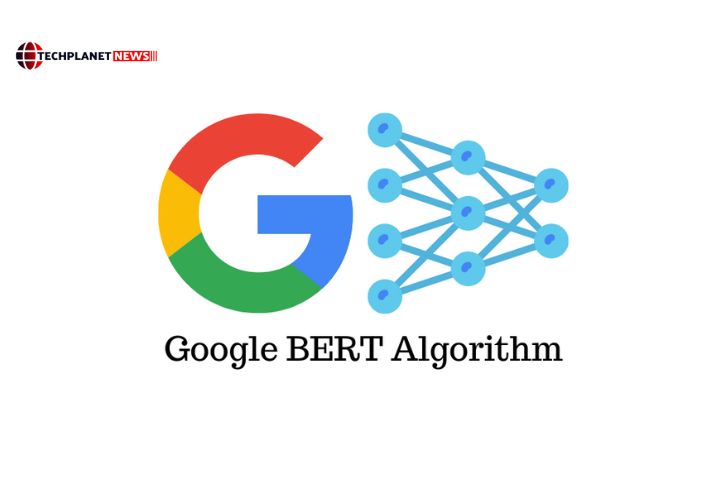
In the ever-evolving landscape of search algorithms, Google has quietly unveiled a groundbreaking framework called TW-BERT that has the potential to reshape the way search results are ranked. The Term Weighting BERT (TW-BERT) framework aims to enhance search ranking accuracy without necessitating major overhauls, making it an intriguing advancement in the field. While Google has not explicitly confirmed its integration into its algorithms, the ease of deployment and significant improvements it offers make TW-BERT a strong contender for adoption.
Below we have discussed the new revolutionizing search; Google’s TW-BERT. Let us see the details for Google’s TW-BERT. This is a new study of Google’s searches from Google Research and the same is being discussed. Check how the same study is going to help you update the new technology for ranking.
Term Weighting BERT (TW-BERT): Bridging Context and Efficiency in Search Ranking
In the realm of search algorithms, innovation is a constant pursuit, and Google’s latest unveiling, Term Weighting BERT (TW-BERT), is poised to redefine the way search results are ranked. This revolutionary framework introduces a novel approach that promises to enhance search accuracy while requiring minimal adjustments to existing systems. While Google has yet to officially confirm its integration into their algorithms, the attributes of TW-BERT make it a compelling contender for a prominent role in the future of search.
Cracking the Code: Unveiling TW-BERT
At the heart of TW-BERT lies a transformative concept – the assignment of scores, known as weights, to individual words within a search query. This groundbreaking approach aims to decode the searcher’s intent by assigning relevance scores to each query term, thereby enabling search engines to deliver more precise and tailored search results.
TW-BERT’s ingenuity extends to query expansion, a process that enriches search queries with additional terms or phrases for improved document matching. By assigning scores to different components of a query, TW-BERT effectively enhances context comprehension, leading to more accurate results that align closely with user expectations.
A Harmonious Convergence: The Marriage of Two Approaches
TW-BERT’s brilliance emerges from its fusion of two distinct search paradigms – the efficiency of statistics-based retrieval methods and the context-awareness of deep learning models. While statistical approaches excel in scalability and domain generalization, they often overlook the contextual nuances of queries. Conversely, deep learning models excel in context understanding but can be intricate to deploy and unpredictable when faced with uncharted territory.
This is where TW-BERT steps in as a mediator, bridging the gap between these two paradigms. By judiciously assigning weights to query terms, it marries the efficiency of existing lexical retrievers with the contextual prowess of deep learning models. In essence, TW-BERT optimizes the weighing process within the retrieval pipeline, leading to improvements in retrieval accuracy while maintaining the consistency of the existing infrastructure.
Unveiling Practicality: A Glimpse into TW-BERT’s Functionality
Consider a search query like “buy keyboard online.” Each word within this query carries specific significance, and TW-BERT understands this intricacy. Take the example of emphasizing the brand “Nike” to ensure that only results containing the desired brand are prioritized. TW-BERT accomplishes this by assigning varying weights to different elements of the query, allowing for precise filtering of results.
Moreover, TW-BERT grasps the contextual relationship between words within a query, such as “keyboard” and “shoes.” According to the higher weight of the phrase “keyboard online” rather than treating the words individually, it strikes a balance between individual word importance and query cohesiveness.
Seamless Integration: TW-BERT’s Appeal for Adoption
An exceptional attribute of TW-BERT is its effortless integration into existing ranking processes. Unlike intricate algorithms that demand complex software or hardware adjustments, TW-BERT serves as a drop-in component, seamlessly enhancing the information retrieval ranking process. This simplicity of integration not only paves the way for potential widespread adoption but also underscores its practicality and effectiveness.
The Unanswered Question: Is TW-BERT Already in Action?
While an official confirmation from Google regarding TW-BERT’s deployment is yet to surface, the framework’s attributes make a compelling case for its potential integration. The combination of easy integration and significant performance enhancements position TW-BERT as a prime candidate for Google’s search algorithm.
The improved ranking accuracy, efficiency, and compatibility with existing systems lend credence to the speculation that TW-BERT could already be driving changes in Google’s search ranking. While we await official confirmation, one thing is certain – TW-BERT is a remarkable innovation that stands poised to redefine the future of search ranking.
Understanding TW-BERT: A Leap Forward in Search Ranking
At the core of TW-BERT lies an innovative approach to ranking search results. This framework assigns scores, or weights, to individual words within a search query to more precisely determine the relevance of documents. This mechanism allows search engines to better comprehend the searcher’s intent and tailor the results accordingly.
A notable feature of TW-BERT is its applicability in query expansion. This process involves refining search queries by adding related terms or phrases, ultimately leading to more accurate matches between queries and documents. By assigning scores to different elements of the query, TW-BERT aids in understanding the context and intent behind each search, resulting in more refined results.
The Bridge Between Two Paradigms: Combining Statistics and Deep Learning
In their research paper, the creators of TW-BERT discuss the convergence of two search paradigms: statistics-based retrieval methods and deep learning models. While statistics-based methods are efficient and scalable, they lack context awareness. On the other hand, deep learning models excel at understanding query context but can be complex to deploy and unpredictable in unfamiliar scenarios.
TW-BERT emerges as a bridge between these approaches, effectively marrying the efficiency of lexical retrievers with the contextual understanding of deep learning models. By assigning appropriate weights to query terms, TW-BERT enriches the search process while maintaining consistency with the existing retrieval pipeline.
The Power of Term Weighting: A Practical Example
Imagine a search query for “buy keyboard online.” Each word in this query requires careful consideration to yield accurate results. For instance, emphasizing the brand is vital to filter out irrelevant brands. TW-BERT comes into play by assigning weights to each component of the query, emphasizing a particular brand while also understanding the context between buying a keyboard and online.
This mechanism ensures that relevant results containing the brand name of the keyboard are prioritized, while also considering the cohesiveness of the query as a whole. By leveraging meaningful n-gram terms and providing appropriate weightings, TW-BERT addresses the limitations of traditional methods.
Seamless Integration: The Key to TW-BERT’s Adoption
One of the standout features of TW-BERT is its ease of deployment. Unlike many other complex algorithms, TW-BERT can be seamlessly inserted into existing information retrieval ranking processes. This straightforward integration allows for potential widespread adoption without requiring major infrastructure changes.
The simplicity of deployment, coupled with the significant enhancements in ranking accuracy and efficiency, makes TW-BERT an appealing candidate for Google’s search algorithm.
The Speculation: Is TW-BERT Already a Part of Google’s Algorithm?
While Google has yet to confirm the use of TW-BERT, its potential inclusion is reasonable to speculate. The framework’s ease of integration and proven effectiveness in refining ranking processes make it a strong candidate for adoption.
TW-BERT’s performance enhancements across different tasks and its compatibility with existing systems contribute to its likelihood of being integrated into Google’s search algorithm. These improvements could very well explain the recent fluctuations in rankings reported by SEO monitoring tools and search marketing experts.
So, this is the study for the new search and one must be understanding how the same will be making changes to help the website rank. This new update is still being discussed and as it is resealed the same will make some changes for the searches we make on the internet. However, if you think the same will not make it difficult for the digital market to implement. There is no such thing with Google’s TW-BERT. This is one of the things that will be taking place to help in the search as we have discussed and provided you some relevant options with examples.
Wrapping up
TW-BERT represents a remarkable step forward in search ranking accuracy. With its ability to seamlessly blend the efficiency of traditional methods with the context-awareness of deep learning models, TW-BERT opens up new possibilities for enhancing the search experience. While Google’s official confirmation remains pending, the signs point to TW-BERT potentially revolutionizing how we navigate the world of search.
We at Tech Planet News make hard efforts to shape the thoughts of our readers. You can comment below to let us know about the same and help with more such information if you want to inform your ideas and be on the top to meet your targeted audience. The information is going to help you with the deep understanding you want to have. Please, let us know how we can provide you with more such information if you want to shape your thoughts with different information.